Automatic Speech Recognition in Natural Language Processing
Natural language processing or NLP gives machines the ability to understand and respond to text and speech in a similar way to human beings. It is a branch of artificial intelligence and a natural language processing company would combine computational linguistics and statistical, machine learning, and deep learning models to give machines this ability to process human language that is presented in the form of text or voice data.
We have all interacted with natural language processing tools in some way or another. Virtual assistants and chatbots use NLP, as do voice-operated GPS systems and speech-to-text software. There are several NLP tasks, which break down text and voice data so that machines can make sense of the data being ingested.
These tasks include grammatical tagging, named entity recognition, and sentiment analysis. Speech recognition is also an NLP task and it is also known as speech-to-text and automatic speech recognition.
Text analytics services will use NLP tasks like speech recognition to convert voice data into text data as seen with virtual assistants, where the human user can give voice commands to the device to request information or use certain functions.
However, speech recognition must take into account various accents, incorrect grammar, and varying pronunciation and emphasis.
Automatic Speech Recognition
Automatic speech recognition or ASR has been described as a cornerstone of the voice experience provided by personal assistants and similar devices. Before the application of the technology, a machine’s understanding of speech was limited to detecting patterns in audio waveforms.
However, with natural language processing and automatic speech recognition, machines can detect these patterns, match them with the sounds in a language and identify the words being spoken. Key components of speech recognition include speech input, feature extraction, feature vectors, a decoder, and word output.
Despite starting by giving machines basic functionality and a limited vocabulary, the technology has today developed to a point where machines can carry out conversations with humans.
With multilingual NLP, for instance, voice services and speech recognition software can understand different languages, accents, and pronunciations. Other techniques and technologies also allow ASR to function accurately at different volumes and with different acoustics and background noise.
This is important as evaluations of ASR tools typically focus on its word error rate (WER) and speed.
Key Features And Algorithms
When using ASR, there are key features that a natural language processing company will focus on. One such feature is language weighting, through which precision is improved by weighting specific words that are spoken frequently.
Profanity filtering is also a part of text analytics services and the ASR feature identifies certain words and phrases with the use of filters.
Speaker labelling is a feature useful in multi-participant conversations, as it adds a transcription that cites or tags each speaker’s contribution. With acoustics training, ASR systems can be trained to adapt to an acoustic environment and speaker styles.
In addition to these key features, algorithms and techniques are used to recognise speech into text and improve transcription accuracy. NLP is one such method used in text analytics services.
N-grams are one of the simplest types of language models and assigns probabilities to sentences of phrases. It is among the main techniques used to improve ASR accuracy and recognition. Speaker diarisation or SD is the process of identifying and partitioning speech by speaker identity. SD algorithms enable machines to better distinguish individual speakers in a conversation.
Hidden Markov models (HMM) are used by a natural language processing company to assign labels to each unit in the sequence, which creates a mapping with the provided input. Neural networks are also an important technique in automatic speech recognition and processes training data by mimicking the interconnectivity of the human brain through layers of nodes. Neural networks are high in accuracy and can accept more data than traditional language models but can be slower.
Applications
Automatic speech recognition is used in different sectors and industries to increase the efficiency and accuracy of services. Customer service, for instance, has improved significantly with the use of ASR as well as natural language processing. Call centres use text analytics services and other AI-powered technologies for sales and customer services and chatbots are an example most are familiar with.
In the healthcare sector, medical transcription tools use automatic speech recognition to accurately capture notes on diagnoses and treatment plans.
Speech recognition has helped improve security protocols as well, with voice-based authentication integrated with security devices. In the automotive industry, speech recognition has been used to enable voice-activated navigation systems.
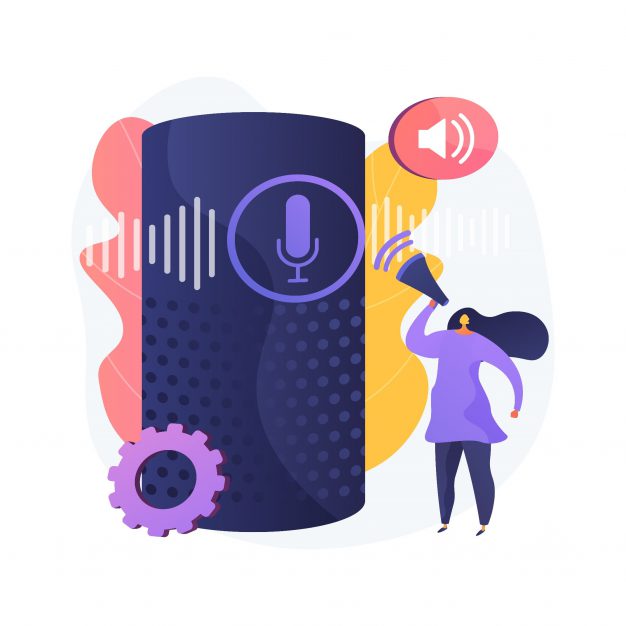